Evolution of Social Media Analytics
From Basic Metrics to Advanced AI Analysis
The evolution of social media analytics reflects a significant transformation in how data is collected, analyzed, and applied. Initially centered around basic metrics, traditional analytics provided a superficial glance at user engagement. As digital platforms grew, so did the complexity of the interactions and the types of data available. The integration of AI has been a game-changer, allowing for the analysis of large data sets rapidly and with more sophisticated outcomes. Now, businesses can uncover not only what content performs well but also why it does, examining the underlying patterns and motivations of user behavior.
Significant Technological Advancements and Their Impact
The integration of technologies such as machine learning and natural language processing (NLP) into social media analytics tools has propelled the capabilities of marketers to new heights. Machine learning algorithms refine themselves over time, learning from the data they process to offer increasingly accurate predictions and insights. NLP has enabled the parsing of human language from posts and comments, facilitating a deeper understanding of consumer sentiments. These technological advancements have provided businesses with the tools necessary to navigate the complexities of social media dynamics effectively and strategically.
Key Components of AI-Driven Social Media Analytics
Machine Learning Algorithms and Their Specific Uses
Machine learning algorithms form the backbone of AI-driven social media analytics. They enable systems to learn from data iteratively and improve over time without being explicitly programmed. These algorithms are essential for identifying trends, segmenting audiences, and personalizing content to enhance user engagement. For instance, clustering algorithms can segment users based on their interaction patterns, while predictive models can forecast future behaviors, providing marketers with a proactive tool to tailor their strategies.
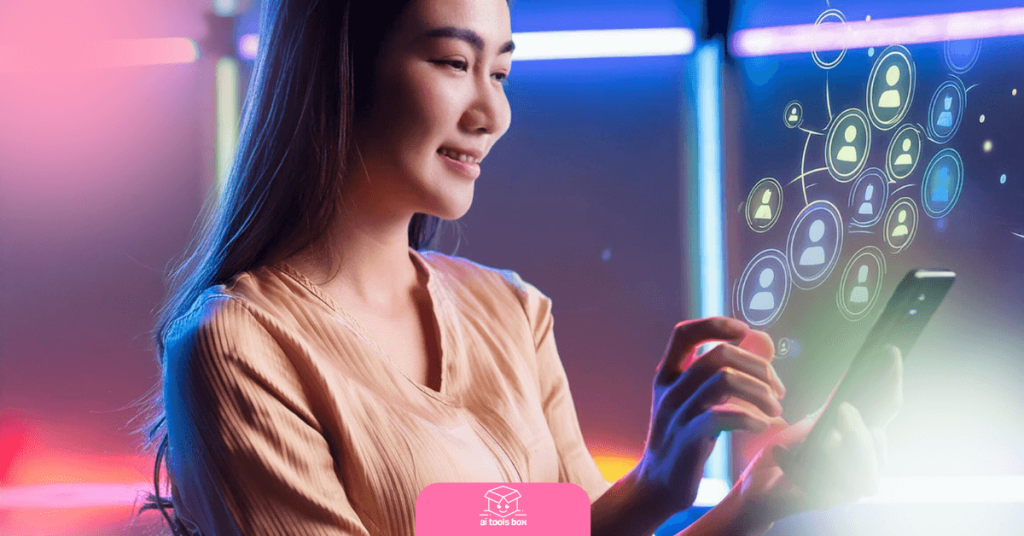
Deep Dive into Natural Language Processing (NLP)
Natural Language Processing (NLP) is another crucial component of social media analytics. NLP technology analyzes the text in posts, comments, and messages to extract meaningful data about public sentiment, trending topics, and customer preferences. This capability allows businesses to understand not just what their audiences are talking about, but also how they feel about various topics and products. Through sentiment analysis, companies can gauge the emotional tone behind social media interactions, which is invaluable for adjusting marketing strategies and improving customer interactions.
Data Mining Techniques and Applications
Data mining in AI-driven social media analytics involves extracting and analyzing vast amounts of data from various social platforms to uncover hidden patterns and insights. Data mining techniques enable the identification of user behavior, content effectiveness, and potential market trends. This process includes collecting data in a structured form, analyzing it with sophisticated algorithms, and generating insights that are actionable and strategically relevant. The application of these techniques allows businesses to stay ahead by making informed decisions based on comprehensive data analysis.
These key components work in synergy to transform raw social media data into strategic insights that drive decision-making and offer a competitive edge in the crowded digital marketplace.
Practical Applications of AI in Social Media Analytics
Detailed Case Studies on AI Implementation
The practical application of AI in social media analytics can be illustrated through detailed, real-world case studies from prominent companies.
Here are two examples:
- Case Study : H&M's Sentiment Analysis for Campaign Adjustments
- Overview: H&M uses sentiment analysis to monitor social media reactions to its fashion campaigns and product launches. By analyzing comments, likes, and shares across various platforms, AI tools assess the emotional tone and sentiment expressed by the audience.
- Implementation: Using a sophisticated AI system, H&M tracks real-time data from social media posts. The system identifies both positive and negative feedback, which allows the marketing team to quickly adjust their strategies. For instance, if a particular product line receives negative sentiment, H&M might boost promotions for more positively received items or address concerns directly.
- Impact: This approach has allowed H&M to enhance customer engagement by being more responsive to consumer needs and preferences. It has led to increased sales during campaigns and has helped H&M maintain a strong brand image by managing potential PR issues effectively.
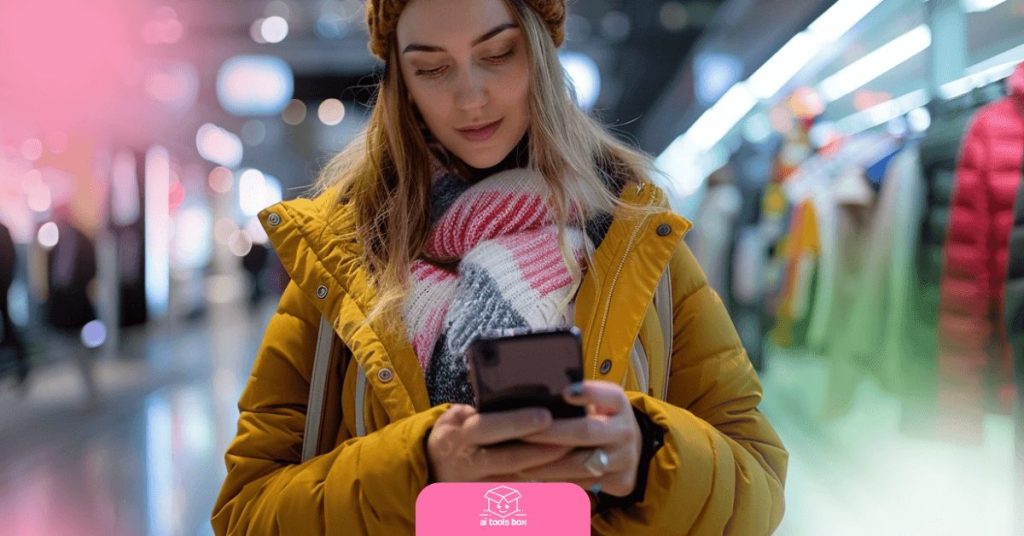
- Case Study : Tesla's Predictive Analytics for Market Trend Identification
- Overview: Tesla leverages predictive analytics to gauge public interest and sentiment towards new technologies and potential product launches, particularly in the realm of sustainable energy solutions.
- Implementation: Tesla’s AI systems analyze social media data to identify emerging trends and discussions around electric vehicles and renewable energy. The technology scans through vast amounts of data to detect patterns and predict which innovations are likely to attract consumer interest.
- Impact: By identifying trends before they fully emerge, Tesla can strategically time announcements and product launches to maximize impact and media buzz. This proactive approach has positioned Tesla as a leader in innovation, directly contributing to its market growth and the strong adoption of its products.
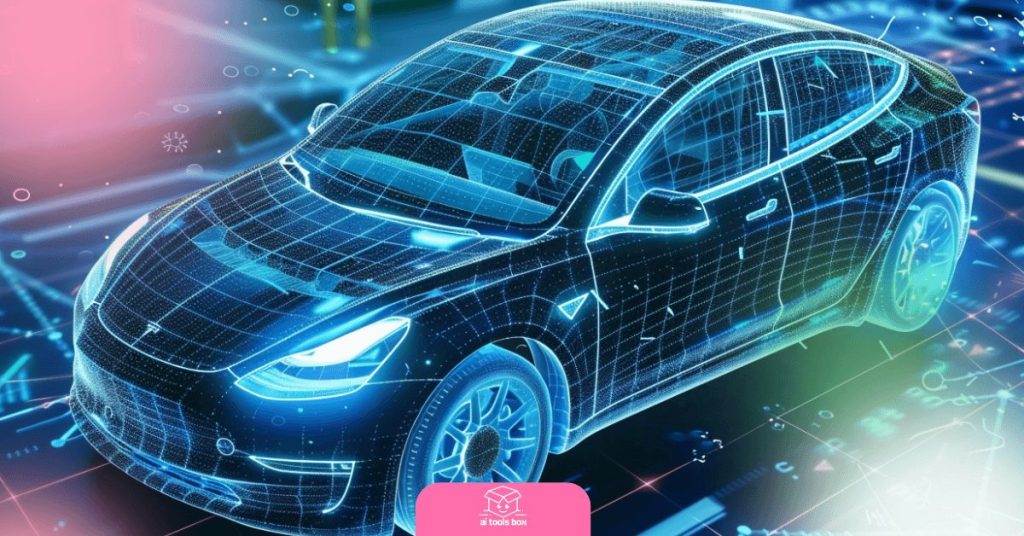
These case studies demonstrate the tangible benefits of AI in social media analytics, highlighting how leading companies utilize these technologies to refine their marketing efforts, respond to consumer sentiments in real-time, and strategically position their products in the market.
Interactive Examples and Visual Aids
To enhance understanding and engagement, interactive examples and visual aids such as infographics and dynamic charts can be integrated into the analytics dashboard. These tools help demystify complex data sets and make the insights more accessible to stakeholders at all levels of an organization. For instance, visualizing sentiment trends over time or mapping the geographic distribution of social media engagement can provide clear, actionable insights that are easy to understand and act upon.
Practical Steps for Businesses to Implement AI
Implementing AI-driven analytics in a business setting involves several practical steps. Initially, companies need to assess their current data capabilities and infrastructure to ensure they can support AI technologies. Following this, partnering with AI technology providers to integrate advanced analytics tools can be crucial. Training is also a key component, as staff must understand how to interpret AI-generated insights effectively. Lastly, it is essential to establish continuous feedback loops to refine AI models and ensure they remain accurate and relevant over time.
These practical applications of AI in social media analytics not only enhance the ability of businesses to understand their audiences but also empower them to act swiftly and efficiently in optimizing their social media strategies.
Ethical Considerations and Challenges
Managing Privacy Concerns and Data Security
In the realm of AI-driven social media analytics, privacy concerns and data security are paramount. Businesses must navigate the delicate balance of leveraging user data for insights while ensuring compliance with global privacy regulations such as GDPR (General Data Protection Regulation). This involves implementing robust data management policies that protect user information from unauthorized access and breaches, and ensuring that data collection and analysis are transparent and consent-based.
Addressing AI Bias and Ensuring Ethical Use
Another significant challenge is the potential for AI bias. Bias in AI models can occur due to skewed training data or flawed algorithms, leading to inaccurate or discriminatory outcomes. To mitigate this, businesses must use diverse data sets and continually audit and update their AI models to ensure fairness and accuracy. Additionally, ethical use of AI involves being mindful of how the insights are used, avoiding manipulative marketing practices and respecting user privacy.
Proposed Industry Guidelines for Ethical AI Practices
To foster trust and sustain long-term engagement, the industry may benefit from adopting standardized ethical guidelines for AI applications in social media analytics. These guidelines could include best practices for data handling, transparency in AI operations, and measures to prevent and address bias. Establishing such standards can help ensure that AI technologies are used responsibly, promoting ethical behavior across all stages of AI implementation.
These ethical considerations and challenges underscore the importance of responsible AI use in social media analytics. By addressing these issues proactively, businesses can not only avoid potential pitfalls but also enhance their credibility and build stronger relationships with their audience.
Impact of AI on Marketing Strategies
Personalization and Targeted Marketing Enhancements
One of the most significant impacts of AI-driven social media analytics is the enhancement of personalization and targeted marketing. With deep insights into audience preferences, behaviors, and interests, businesses can craft highly personalized marketing campaigns. These tailored approaches are more likely to resonate with specific audience segments, leading to higher engagement and conversion rates. For instance, by analyzing user interaction data, companies can create content that aligns perfectly with the interests of different demographics, increasing the relevance and effectiveness of their marketing messages.
Predictive Analysis and Future Trend Forecasting
Predictive analysis is another critical advantage offered by AI in social media analytics. This technique utilizes historical data and current trends to forecast future behaviors and market demands. Such insights allow businesses to anticipate market changes, adapt their strategies accordingly, and stay ahead of their competitors. Predictive analytics also plays a crucial role in risk management by identifying potential issues before they become significant problems, enabling proactive rather than reactive strategies.
Case Examples of Strategic Transformations Through AI
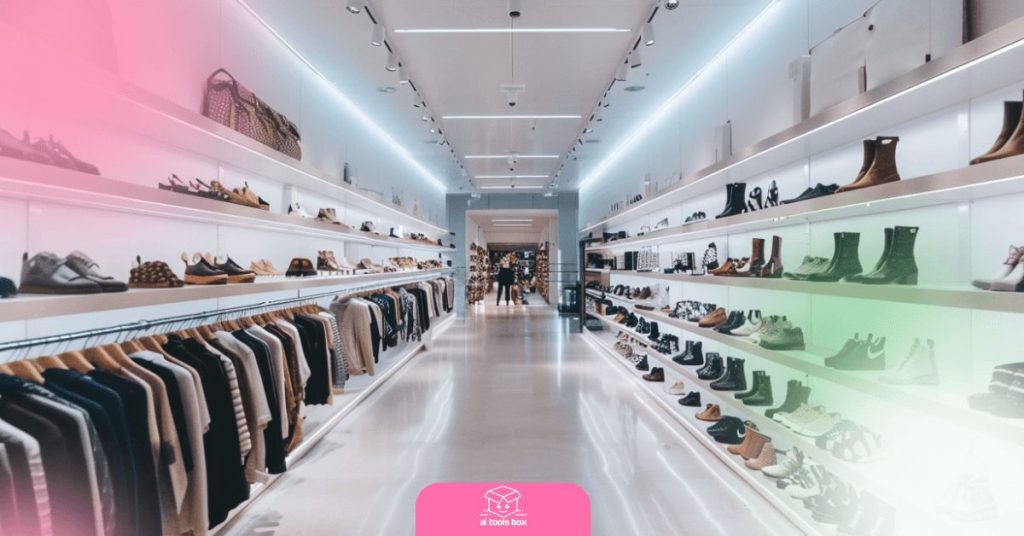
To illustrate the impact of AI, consider the case of a fashion retailer that uses trend forecasting algorithms to determine which styles are likely to become popular. This information enables them to adjust their inventory and marketing strategies in advance, capitalizing on trends as they emerge.
Another example is a tech company that uses sentiment analysis to gauge reactions to a new product launch, allowing them to quickly address customer concerns and improve their offerings.
These strategies highlight how AI-driven analytics not only supports more informed decision-making but also transforms marketing approaches from generalized broadcasting to strategic, insight-driven engagement. The ability to harness and apply these insights effectively is becoming a critical differentiator in the competitive landscape of digital marketing.
Examples of companies effectively utilizing AI-driven social media analytics to enhance their marketing strategies and overall business operations:
- Coca-Cola’s Social Media Sentiment Analysis
- Overview: Coca-Cola uses AI-driven sentiment analysis to monitor social media reactions to their products and campaigns. This technology helps them gauge public sentiment and quickly respond to customer feedback.
- Impact: The insights gained from sentiment analysis have informed several marketing decisions, including campaign adjustments and new product launches, helping to maintain a positive brand image and increase consumer engagement.
- Airbnb’s Machine Learning for Dynamic Pricing
- Overview: Airbnb employs machine learning algorithms to help hosts optimize their pricing based on various factors such as location, seasonality, and local demand.
- Impact: This AI capability allows hosts to maximize their earnings and improves the platform’s competitiveness by offering more attractive pricing to guests. It also enhances user satisfaction by ensuring fair pricing reflective of real-time market conditions.
- Zara’s Use of AI for Trend Forecasting and Inventory Management
- Overview: Zara utilizes AI to analyze real-time sales data and customer preferences across its global network of stores to predict future fashion trends.
- Impact: This predictive insight helps Zara to manage inventory more efficiently, reduce overstock situations, and ensure that popular items are adequately stocked. As a result, Zara maintains its reputation for having a quick turnaround in trend adoption and keeping customer satisfaction high by having desired products available.
- American Express’s Fraud Detection Algorithms
- Overview: American Express uses AI algorithms to detect potential fraudulent transactions in real time, analyzing patterns that deviate from typical customer behavior.
- Impact: This proactive fraud detection not only prevents financial loss but also protects customer accounts, enhancing trust and security across its services. The speed and accuracy of these AI systems are crucial for maintaining customer confidence in a highly competitive financial services market.
- Unilever’s AI-Driven Market Research
- Overview: Unilever utilizes AI for deep learning analysis of social media data to understand consumer needs and emerging trends.
- Impact: This approach allows Unilever to stay ahead of market trends and quickly adapt their product development and marketing strategies. By aligning closely with consumer preferences discovered through AI analytics, Unilever can more effectively meet customer demands and strengthen brand loyalty.
These cases showcase how diverse industries leverage AI in social media analytics not just for enhancing marketing efforts but also for improving operational efficiencies and customer relationships.
Future of AI in Social Media Analytics
Predictions for Emerging Technologies
As AI technologies continue to evolve, the future of social media analytics is poised to be influenced by several key advancements. One significant area of development is the integration of augmented reality (AR) and virtual reality (VR). These technologies could revolutionize the way brands interact with consumers on social media, providing more immersive and engaging experiences. Additionally, the improvement of voice and visual search capabilities will expand the types of data available for analysis, requiring more sophisticated AI models to interpret these new data streams effectively.
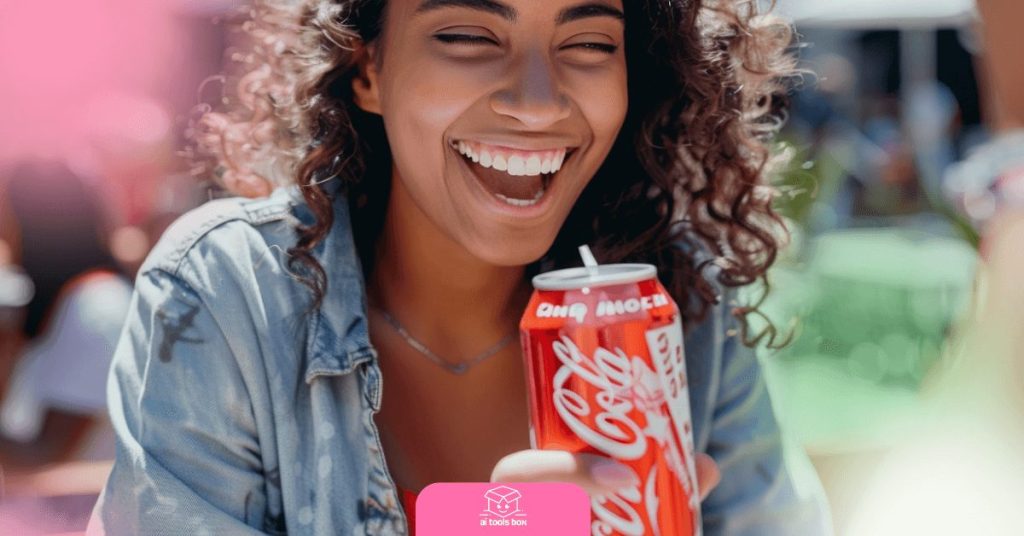
The Role of Advanced Computational Technologies
The potential integration of quantum computing in AI analytics could drastically increase the speed and accuracy of data processing, allowing for near-instantaneous insights into large data sets. This could enhance predictive analytics, making it possible to achieve more accurate forecasts of consumer behavior and market trends. Moreover, the continued advancement of machine learning models, especially in unsupervised learning, is likely to enable more nuanced and complex analysis without human oversight.
Expert Opinions and Industry Reports
Leading industry experts predict that AI will become even more integral to business strategies. Companies that can adapt to AI-driven analytics will likely find themselves at a competitive advantage, with the ability to not only react to market changes but also anticipate them. Industry reports suggest that businesses will increasingly rely on AI to conduct cross-platform analytics, providing a holistic view of consumer interactions across all digital mediums. This comprehensive approach will allow for more coherent and unified marketing strategies that are seamlessly integrated across all channels.
In summary, the future of social media analytics is expected to be characterized by more sophisticated AI applications, offering greater personalization, predictive power, and cross-platform integration. These advancements will enable businesses to not only keep pace with the rapid evolution of digital marketing but also to stay ahead of emerging trends, providing them with the tools necessary to innovate and thrive in an increasingly complex marketplace.
Conclusion and Call to Action
As we have explored throughout this discussion, AI-driven social media analytics has significantly transformed how businesses interact with and understand their audiences. The integration of AI has enabled more precise, real-time insights, allowing companies to craft personalized marketing strategies, anticipate market trends, and enhance customer engagement effectively.
Summary of AI's Transformative Impact
The transformative impact of AI in social media analytics is clear. It has shifted the marketing landscape from a reactive to a proactive approach, where businesses are not just responding to consumer behaviors but anticipating them. From improving personalization to enhancing predictive analytics, AI has provided tools that enable deeper and more meaningful connections with customers.
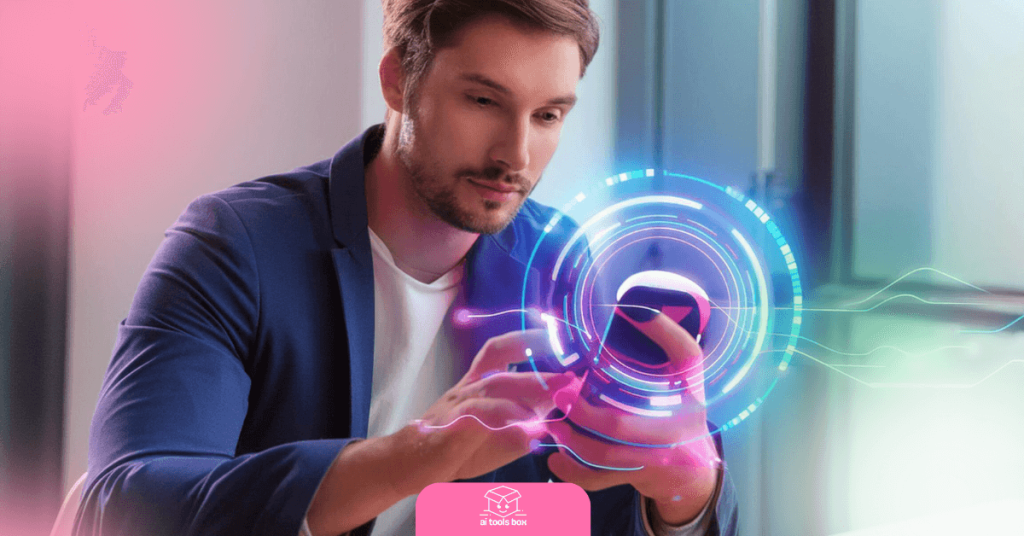
Encouragement to Adopt AI Tools
For businesses looking to remain competitive in the dynamic and ever-evolving digital marketplace, adopting AI-driven analytics is no longer just an option but a necessity. The insights gained from AI can help businesses make informed decisions, tailor their marketing efforts more effectively, and ultimately achieve a greater return on investment.
Resources and Support for AI Implementation
To facilitate this transition, businesses should consider partnering with technology providers who can offer the necessary tools and expertise. Additionally, investing in training for their teams to understand and utilize these tools effectively will be crucial. For those unsure of where to start, many industry organizations offer resources and workshops on implementing AI in business practices.
Call to Action:
We encourage businesses to embrace these technological advancements and start integrating AI into their social media analytics practices. By doing so, they will not only enhance their marketing strategies but also strengthen their customer relationships and secure a competitive edge in their industry. The future of marketing is data-driven, and with AI, that future is now.
In conclusion, as the digital landscape continues to grow and evolve, AI-driven social media analytics will play an increasingly vital role in shaping successful business strategies. Now is the time to act, adapt, and advance with AI.